Joint VU/Leiden/Delft seminar on the 2nd of April
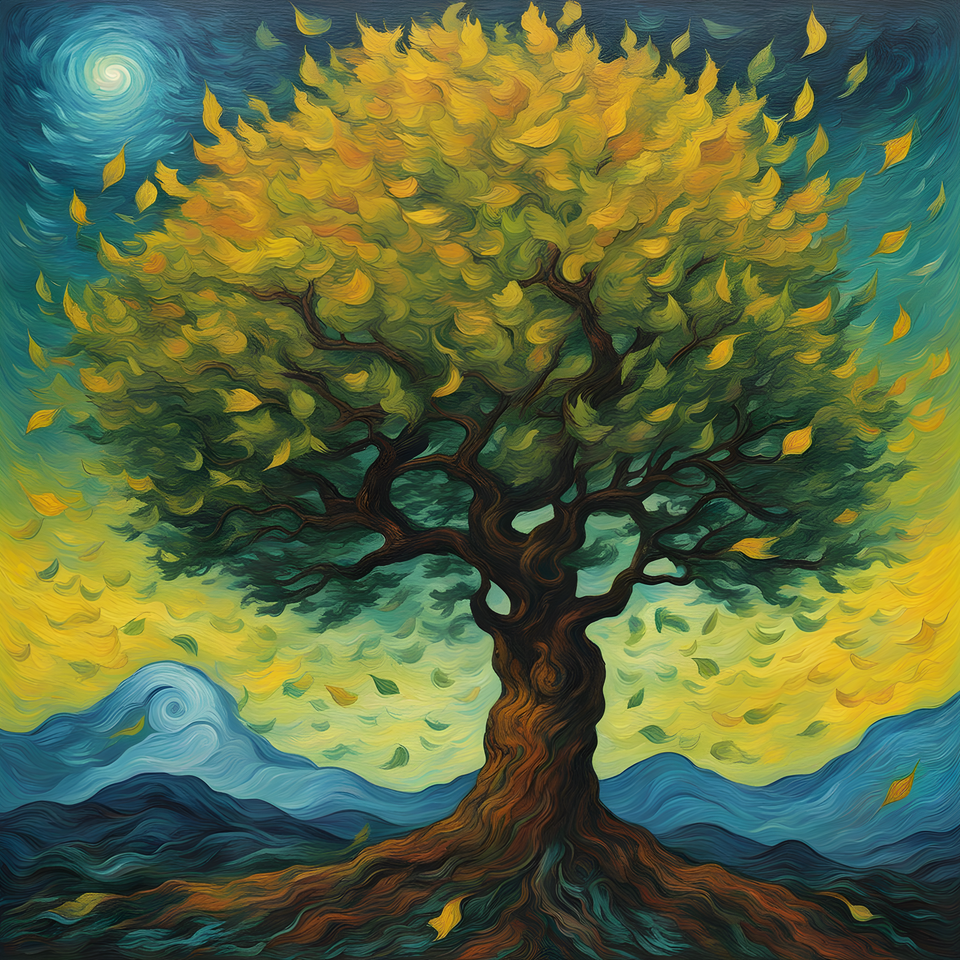
The joint VU/Leiden/Delft seminar is a series of one-day events taking place twice a year, and organised by Svetlana Dubinkina (VU), Frits Veerman (LU), and Yves van Gennip (TU Delft).
The next seminar will take place on Wednesday the 2nd of April at VU Amsterdam, in room 9A.46 of the NU-VU building.
Registration is available here and it is required for catering purposes.
Preliminary programme, 2 April 2025
10:00 - 10:30 Walk in and coffee
10:30 - 11:30 Arjen Doeman (Leiden)
11:30 - 12:00 PhD students Q&A / coffee break *
12:00 - 13:00 Lindsay van der Aalst (VU Amsterdam)
13:00 - 14:00 PhD students Q&A / lunch *
14:00 - 15:00 Sjoerd Dirksen (Utrecht)
15:00 - 15:30 PhD students Q&A / tea break *
15:30 - 16:30 Victorita Dolean-Maini (TU Eindhoven)
16:30 PhD students Q&A / drinks
*During the PhD students Q&A, the PhD students will get an opportunity to interact with the speakers without any other senior mathematicians present, who will be having their coffee break outside the room.
Speaker: Arjen Doelman
Title: The dynamics of patterns in spatially inhomogeneous reaction-diffusion systems
Abstract: Mathematics may provide crucial insights in the evolution of ecosystems under varying circumstances (for instance induced by climate change), vice versa, studying ecosystem dynamics challenges and stimulates the development of novel mathematical theories. Ecosystem models typically are of reaction-diffusion type, with components representing (various kinds of) vegetation, soil and overland water, etc.. The local topography of the terrain is an important factor, and it appears as a heterogeneous spatially varying term in such models. However, the mathematical theory of pattern formation in inhomogeneous systems is at present still at its infancy. In this talk we first briefly consider earlier studies of two-component reaction-diffusion models that focus on the persistence under a heterogeneous effect of (singular) localized structures that exist (and are stable) in the homogeneous limit. To build a conceptual understanding of the impact of such a heterogeneity on the dynamics of patterns exhibited by a reaction-diffusion model, we next consider the most simple setting of a scalar bi-stable Allen-Cahn model driven by a small spatially heterogeneous effect. Motivated by the observation that the stationary problem associated to the PDE can be seen as a (weakly) driven planar Hamiltonian system, we consider the question as, “What kind of novel (stable) multiple-front patterns may be initiated by the heterogeneous topographical effects?”, and “What is the impact of the heterogeneity on the dynamics of (multiple) interacting patterns/fronts?” In the case of a spatially periodic topography these questions bring us into the realm of (spatial) horseshoe dynamics, for spatially localized topographies we will show that the decay rates of the topography have a crucial impact on the long-term dynamics of multi-front patterns. (This is joint work with Robbin Bastiaansen & Tasso Kaper.)
Speaker: Lindsay van der Aalst
Title: Computer-assisted proofs for periodic traveling waves in higher dimensional partial differential equations
Abstract: The one-dimensional suspension bridge equation has been well studied: traveling waves of various types have been proven to exist. Far less is known about the two-dimensional case. We use computer-assisted proof methods to find and prove periodic traveling waves in two spatial dimensions, ensuring rigorous control over computational errors. Additionally, we extend computer-assisted proof techniques from existing literature on two-dimensional periodic solutions in the forced Navier-Stokes equations to three dimensions to prove traveling wave solutions. Challenges posed by the higher-dimensional nature of the problem are alleviated by employing symmetry arguments.
Speaker: Sjoerd Dirksen
Title: The separation and memorization capacities of neural networks
Abstract: In this talk, I will first consider the separation capacity of neural nets - the ability to transform two classes (with positive distance) into linearly separable ones. I will show that already sufficiently large two-layer ReLU nets with random weights and biases, which arise as the initialization of ReLU nets, have this capacity with high probability. In the second part of this talk I will build on the geometric insights behind this result to shed new light on the memorization capacity of neural nets - their ability to perfectly fit given labeled data. I will present a simple randomized algorithm to produce a small interpolating neural net for given data with two classes. This method yields new memorization capacity results for neural nets that move beyond worst case lower bounds in the literature. In both results, the sufficient size of the network is linked to new complexity measures that describe both the geometric properties of the two classes and their mutual arrangement.
Based on joint works with Patrick Finke (UU), Martin Genzel (UU, Merantix Momentum), Paul Geuchen (Eichstätt-Ingolstadt), Laurent Jacques (UC Louvain), Alexander Stollenwerk (UC Louvain)
Speaker: Victorita Dolean-Maini
Title: Accelerating Computations of Waves in Complex Media: From Classical Domain Decomposition Solvers to Learning
Abstract: Wave propagation and scattering problems are of huge importance in many applications in science and engineering - e.g., in seismic and medical imaging and more generally in acoustics and electromagnetics. Large scale simulations of those applications are one of the hard problems from computational point of view since requires an interplay between the parsimonious but sufficiently accurate discretisation methods and more sophisticated solution methods. Our aim is to show on one side, how classical domain decomposition methods developed in the latest years coupled with carefully chosen discretisations can help in this endeavour. On the other side, we would like to propose some openings towards the very attractive package of approximation-solution-optimisation offered by the new methods in scientific machine learning.